Essential AI terminology: What you need to know
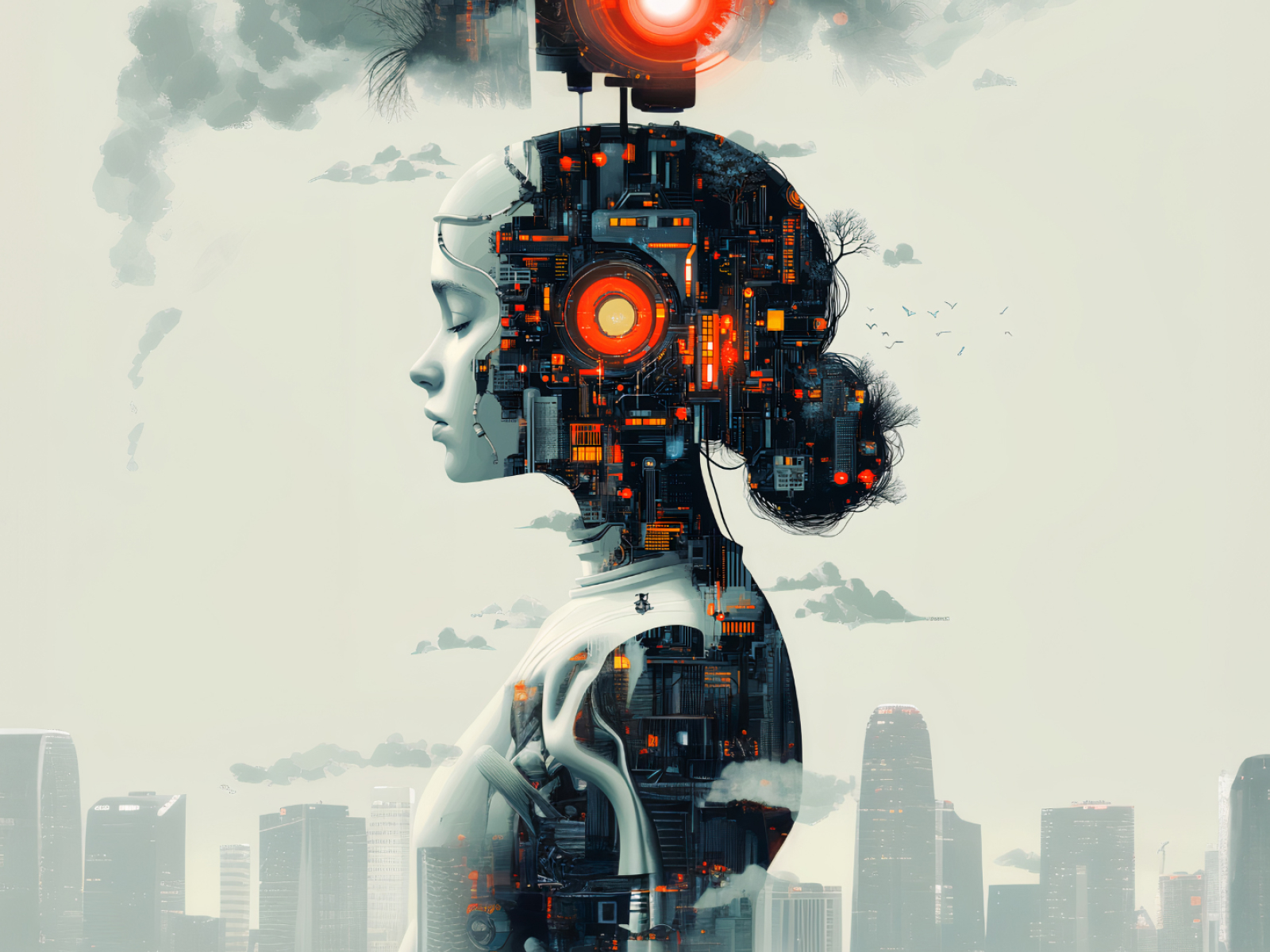
Whether you're diving into Artificial Intelligence I for the first time or looking to deepen your understanding, knowing the right terminology is crucial for navigating this dynamic field. From machine learning to neural networks, here’s a breakdown of important AI concepts you need to know.
Artificial Intelligence (AI): At its core, AI is a broad field that aims to create machines that mimic human intelligence. This includes everything from a simple chess program to a complex system that can understand and respond to natural human language. Remember, AI is not just about replicating human intelligence, but also about complementing it.
Algorithm: An algorithm is a set of rules or a step-by-step process that a computer follows to solve a problem or complete a task. In the context of AI, algorithms are the foundation of the models used for tasks like learning, prediction and optimization. They guide the machines through the process of finding patterns, making decisions and improving their performance.
Machine Learning (ML): Often used interchangeably with AI, Machine Learning is actually a subset of AI. It involves teaching machines to learn and adapt from experience, just like humans do. Think of it as a student who learns from textbooks (data) and then is able to solve problems (make predictions) based on that knowledge.
Neural Network: A neural network is a series of algorithms that identifies underlying relationships in a set of data. These networks can learn and improve over time, and are the backbone of deep learning.
Deep Learning (DL): This is a further subset of Machine Learning, inspired by the structure and function of the human brain (specifically neural networks). Deep Learning algorithms attempt to draw similar conclusions as humans would by processing data through layers of artificial neural networks.
Supervised Learning: This is a type of Machine Learning where the model is given labeled training data and the goal is to learn a mapping from inputs to outputs. It's like a teacher supervising the learning process. The model learns from the labeled data, while the teacher guides the learning process, ensuring the model gets closer to the correct output.
Unsupervised Learning: In contrast to supervised learning, unsupervised learning involves training a model using no labels at all — it’s like learning without a teacher. The model is left to find patterns and relationships within the data on its own.
Natural Language Processing (NLP): Ever wondered how Siri or Alexa understand your voice commands? It's all thanks to NLP. This field of AI focuses on the interaction between computers and humans through natural language. The ultimate objective of NLP is to read, decipher, understand and make sense of human words in a valuable way.
Computer Vision: This term refers to the ability of machines to visually interpret images, often in more detail than the human eye. It's the technology behind self-driving cars, facial recognition software, and other technologies that extract rich information from images or video.
Generative AI: This is a type of artificial intelligence, better known as GenAI, can create content. Think of it as an imaginative artist but in the form of an AI model. Whether it's a piece of writing, an image, or even a piece of music, generative AI can produce them from scratch. It does this by learning patterns from large amounts of data, and then uses this understanding to generate creative output. This technology is behind many of the AI-powered projects you might see today, like AI-written articles or AI-generated art.
Large Language Model (LLM): Large Language Models are deep learning models with billions of parameters, and are much larger than typical neural networks. They can be used for many different applications, including classification and text generation, with surprisingly accurate and human-like results. Large language models are expanding our capabilities with AI-powered tools like chatbots, translation services and content generation systems.
About unitQ
unitQ revolutionizes how product builders, engineers, support leaders and team members understand feedback in real time to build superior products, fix bugs faster and resolve support issues at scale. With unitQ’s customer feedback platform, you can discover quality issues at the same time as your users; know what product launches, releases or evergreen features are causing the most bugs or support tickets; or drill into the root causes of these issues by source, platform, device, customer segment and more.
unitQ AI centralizes feedback from all feedback sources and automatically groups it into thousands of granular categories to help organizations discover what matters most to users — all in real time. Customer-centric companies like Spotify, Bumble, Pinterest, DailyPay and Zendeskrely on unitQ for actionable insights to drive growth, reduce churn and build brand loyalty.
Want to see how your organization compares to others? Get your free unitQ Score or book a unitQ demo today!
David Kravets is Senior Content Marketing Manager at unitQ.